By Francie Fink
As of 2020, almost a third of American households reported difficulty in paying energy bills, or were forced to keep their home at an uncomfortable and potentially unsafe temperature to save money. With increasing summer temperatures and more extreme winter temperatures anticipated in years to come, this statistic is likely to become only more alarming. Policymakers and researchers have developed a metric to standardize which homes pay more than others towards energy bills, as a proportion of their annual household income. That metric, called a household’s “energy burden” can be calculated and expressed as a simple percentage:
(Annual money spent on energy costs)/(Annual household income) * 100.
You might now easily calculate your own household’s energy burden. You might also easily see how this proportion can be alarming: for instance, for a household making $25,000 a year with $2,000 towards energy bills in that same year, that family’s energy burden is 8%. Common standard is that 6% energy burden or higher constitutes an energy-poor household, this being the average energy burden of a household between 0–80% area median income (AMI). A tricky characteristic of this calculation for policymakers hoping to intervene is that we often don’t have accurate, accessible data on household income. This component is key in accurately estimating household energy burden and is essential for effectively directing assistance to those who need it most.
Minnesota can experience bitterly cold winters and, thus, its households rely on dependable heating. Energy bills can rack up for low-income households, particularly for those heating with expensive fuels and inefficient equipment. In the Twin Cities, for households less than 30% AMI, the average household energy burden for Minneapolis is 10%—and for St. Paul, 7%. While incoming Inflation Reduction Act funding and existing utility programs provide an opportunity for energy-efficient upgrades and bill assistance, respectively, it’s currently very difficult for administrators to know which households can benefit most. If a family is seeking assistance through Minnesota’s Energy Assistance Program, as of now, they must first find that the program exists, then fill out a somewhat lengthy application with an income-verification step. Or, if program administrators aren’t able to reach target households, these households might instead engage in risky financial behavior, such as taking on loans to keep the heat on.
A small group of researchers at the University of Minnesota’s (UMN) Center for Sustainable Building Research partnered with the Midwest Big Data Innovation Hub (MBDH) to improve the methodology of estimating household income in an effort to enable proactive outreach to families experiencing high energy burden.
“Energy burden is often addressed as something that has to have already been experienced,” says Diba Malekpour Koupaei, Research Fellow at the Center for Sustainable Building Research. “We aim to be proactive by keeping an eye out for households that may currently be experiencing it or could face this challenge in the future. This approach has the potential to increase our capacity for outreach to those most in need of intervention.”
The team, led by Malekpour, aimed to develop a framework for estimating household income using what is called the House Price-to-Income Ratio. The metric is essentially exactly what it sounds like, and its main purpose is to gauge the affordability of a home for a given family. The UMN group realized they might be able to use this metric for something else: income estimation in the context of energy burden. The value of a home might just be a good predictor of its occupants’ income.
Researchers can and frequently do use microdata, or unidentifiable, individual-level data, from open sources like the U.S. Census’ American Community Survey (ACS). The ACS has information on income, home value, and energy costs for a sample of American homes, so it can be valuable for energy-burden studies. Previous research by the UMN and other groups couldn’t identify a correlation between household income and home value within the dataset. Building on work from another study, however, the UMN team was able to use a relationship between home value and income when matching up deciles of these two metrics.
The relationship between household income and home value wasn’t a strong enough predictor when analyzing individual data points. But when the entire distribution of home values was matched on a decile basis to the distribution of incomes, a significant relationship revealed itself. When both datasets are split into equal 10% parts, a linear regression model can be established for each matched pair of deciles. For example, the lowest 10% of home values are compared to the lowest 10% of incomes, and so on up to the highest deciles. This shows the relationship between household income and home value more effectively than traditional methods.
Through some trial and error, the UMN was able to use the decile-to-decile approach to establish a relationship that can be used across the country, not just in Minnesota. In practice, this relationship can be used to estimate income at the Census block level, when geomatched with local home assessment data. Using the 6% threshold for the amount of earnings a household can spend on energy costs before becoming overburdened, the research team was able to take their findings one step further. Solving for “amount of money spent on energy costs annually” in our original energy-burden equation under 6% energy burden and a given income, one can find the maximum amount of money a household is able to spend yearly before becoming overburdened. The graph below shows that, interestingly, different neighborhoods in Minneapolis have noticeably different annual energy budgets. See, for instance, that single-family homeowners in downtown and uptown communities can spend more on energy each year before being overburdened than the Powderhorn and Phillips communities. The same, though, might not be true for those in multifamily housing.
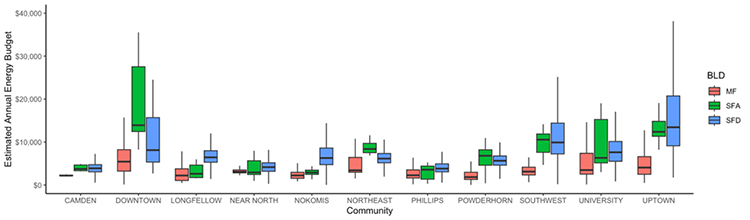
The MBDH supported Malekpour and her team’s research in 2024. The team’s work has resulted in a methodology manuscript currently in submission. The publication will come up in a lookup table and can be used anywhere as long as there is assessor data available. The assessor data will serve as a broadly available source for home value in determining the relationship to household income. The team hopes to make their methodology publicly available, potentially changing the game for energy-assistance and home-upgrade programs seeking out families vulnerable to high energy costs in relation to their earnings.
Also in the works is an interactive geographic information system (GIS) interface that uses the methodology to convey household energy budgets on a regional or even more granular scale. The interface would allow individuals to contribute their own income, home-value, and energy-cost data anonymously to hone the model. The UMN team hopes to recruit graduate students to incorporate this voluntary data in the near future.
Get Involved/Learn More
Learn more about the UMN Center for Sustainable Building Research here.
Contact the Midwest Big Data Innovation Hub if you’re aware of other people or projects we should profile here, or to participate in any of our community-led Priority Areas. The Midwest Big Data Innovation Hub is an NSF-funded partnership of the University of Illinois at Urbana-Champaign, Indiana University, Iowa State University, the University of Michigan, the University of Minnesota, and the University of North Dakota, and is focused on developing collaborations in the 12-state Midwest region. Learn more about the national NSF Big Data Hubs community.