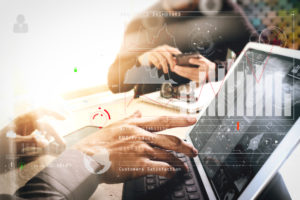
AI and open source have emerged as essential tools for businesses seeking to enhance efficiency and drive innovation. But, how do two transformative forces intersect and impact the data science community? They surely offer new opportunities for data science, but there is also a sense of unreadiness in tackling emerging tools and addressing critical issues like security concerns.
Despite the challenges, adoption continues to surge. An overwhelming majority (87%) of data science practitioners are spending more time or as much time on AI techniques compared to last year, according to a new report by Anaconda. The AI techniques include using generative adversarial networks (GANs), deep learning, and transformer models.
However, about one in four respondents (26%) said their companies have an interest in AI but don’t have the budget or support to drive business value. In addition, 43% of respondents feel unprepared to handle data science challenges such as government regulations, an increase in AI usage across roles, and the steep learning curve for some technology tools.
Just 22% of respondents fear AI will take their jobs, a steep decline from last year’s report. This reveals that fewer people are concerned about AI overtaking their jobs. Instead, they are weaving AI into their existing workflows, using it to handle laborious or repetitive tasks. This allows them to concentrate on more innovative and high-level pursuits.
According to the report, the top use cases of AI include data cleaning, visualization, and analysis (67%), automating tasks (52%), and prediction or detection models (52%).
The top benefits of open-source software include speed of innovation, cost-effectiveness, and the flexibility for developers to tailor solutions to specific project needs. While open source and AI bring value, they also come with some unique challenges, with security being a chief concern.
Open-source security was cited as the biggest technical challenge for AI adoption and usage (42%). This might be because open-source code is transparent and accessible, which can make it an easy target for malicious actors.
The findings are part of the 7th Annual Data Science Report: AI and Open Source at Work which is based on a survey of over 3000 professionals from 136 countries. The respondents included data science practitioners, IT workers, students, and researchers or university professors.
In this year’s report Anaconda, a provider of data science, machine learning, and AI solutions, focused on the latest trends across the data science, AI, and open-source community.
“AI innovation doesn’t happen in isolation. The collaboration of passionate communities fuels it,” said Peter Wang, Chief AI and Innovation Officer at Anaconda. “To make that collaboration work, data scientists and developers need tools that offer secure scalability and reliable governance controls.”
Wang then emphasized how open discussion and shared problem-solving reinforce these collaborative efforts. “Beyond these tools, data scientists and developers also need open channels for sharing insights, raising concerns, and collectively solving problems,” he continued.
“When organizations support these collaborative ecosystems, internally and across the broader open-source community, they create fertile ground where innovation thrives and challenges like security can be tackled head-on.”
Regulations for AI remain a lingering concern for data scientists. This includes the need to ensure the explainability and transparency of AI models (38%), addressing bias and fairness in AI algorithms (36%), and facilitating collaboration between academia and industry (14%).
Anaconda emphasizes in the report that collaboration is key to addressing some of these challenges. It recommends that the data science community should encourage and support learning, open dialogue, and collaboration internally and within the larger data science ecosystem.
“Having established processes internally with a really strong sense of what ‘good’ looks like is very important,” shared Greg Jennings, VP of Engineering for AI, Anaconda. “If you don’t have an internal way to evaluate the quality of the response, it’s going to be difficult for you to apply AI to it effectively. So much about applying AI to any problem is understanding how you iterate the system to get an increasingly better-quality answer.”
The report highlights that AI and open source function best when collaboration is involved. However, 34% of IT administrators don’t feel empowered to voice their concerns about security risks related to AI and open-source tools.
Along with collaboration, Anaconda recommends supporting education and teaching to nurture the workforce through these early phases of the AI technological shift. Data science practitioners and IT respondents share that online courses, workshops, and in-person training programs are the best methods for educating and teaching. These can be complemented by peer learning and mentorship programs. Collaboration, communication, and continuous learning are highlighted by Anaconda as vital ingredients for deriving maximum value from AI and open-source tools for data science.
Related Items
OSI Open AI Definition Stops Short of Requiring Open Data
IBM Unveils New Open Source Granite Models to Enhance AI Capabilities
Beyond the Moat: Powerful Open-Source AI Models Just There for the Taking