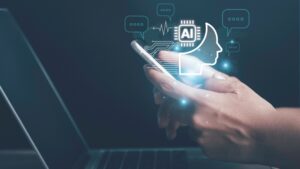
Monte Carlo today rolled out a pair of AI agents designed to help data engineers automate tough data observability problems, including developing data observability monitors and drilling into the root cause of data pipeline problems.
Monte Carlo has made a name for itself as one of the preeminent data observability tool providers. While the company uses machine learning algorithms to detect data pipeline anomalies, its offerings have traditionally leaned heavily on the expertise of human data engineers and data stewards to understand the context of data and data relationships.
That is starting to change with the introduction of agentic AI capabilities into the Monte Carlo offering. Today, the company announced two observability agents, including a Monitoring Agent and a Troubleshooting Agent, that it claims will dramatically speed up time-consuming tasks that previously were dependent on human expertise.
For example, the new Monitoring Agent will allow customers to create data observability monitors with thresholds that make sense for the particular environment that it’s being deployed in. That previously required the diligent work of a data engineer or data steward to create thresholds that were neither too noisy nor too permissive.
Finding that Goldie Locks zone used to take humans, but it can now be done reliably with agentic AI, says Monte Carlo Field CTO Shane Murray.
“That usually requires a lot of business context, requires a lot of understanding of the data and of the business to be able to create these rules and to define useful alert thresholds,” Murray tells BigDATAwire. “What the monitoring agent does is it identifies sophisticated patterns across columns in the data, across relationships, and essentially profiles both the data to understand how it correlates and what are the potential anomalies that can occur in the data; the metadata to understand the context for how it’s used; and then query logs to understand the business impact of those. And then it suggests to the user a series of recommendations.”
Monte Carlo had already started to dabble with agentic AI. In late 2024, it gave customers the ability to have generative AI suggest monitoring rules, which is what became the Monitoring Agent. The company has several customers already using this offering, including the Texas Rangers baseball team and Roche the pharmaceutical company. Together, these early adopters have used the GenAI to create thousands of monitor recommendations, with a 60% acceptance rate.
With the rollout of the Monitoring Agent, the company is taking the next step and giving customers the option of putting these observability monitors into production, albeit in a read-only manner (the company isn’t letting AI make any changes to the systems). According Lior Gavish, the CTO and co-founder of Monte Carlo, the Monitoring Agent increases monitoring deployment efficiency by 30 percent or more.
The Troubleshooting Agent, which is currently in alpha and currently scheduled to be released by the end of June, goes even further in automating steps that previously were done by human engineers. According to Murray, this new AI agent will spawn multiple sub-agents to fan out across multiple systems, such as Apache Airflow error logs or GitHub pull requests, to look for evidence of the cause of the data pipeline error.
“What the troubleshooting agent does is it actually tests a number of these hypotheses about what could have gone wrong,” Murray says. “It tests it in the source data. It tests it across potential ETL system failures, various code that have been checked in.”
There could be hundreds of subagents spawned that will all work in parallel to find evidence and test hypothesis about the problem. They will then come back with a summary of what they found, at which point it’s back in the hands of the engineer. Monte Carlo says early returns indicate the Troubleshooting Agent could reduce the time it takes to resolve an incident by 80%.
“I see this as going from root cause analysis to being very manual and essentially taking days or weeks down to a state of us giving you the tools so you could potentially do it in hours,” Murray says, adding that it’s essentially “supercharging the engineer.”
With both of these agents, Monte Carlo is trying to replicate what human workers would do by analyzing data and then taking appropriate next steps. Monte Carlo is looking for additional AI agents to build to further streamline data observability for customers.
The two AI agents are based on Anthropic Claude 3.5 and run entirely in Monte Carlo’s environment. Customers do not need to set up or run a large language model or pay an LLM provider to make use of them, Murray says.
Related Items:
Will GenAI Modernize Data Engineering?
Monte Carlo Brings GenAI to Data Observability
Monte Carlo Detects Data-Breaking Code Changes